Discovering a Local Fluctuation Theorem with Machine Learning
© The Physical Society of Japan
This article is on
Machine learning a time-local fluctuation theorem for nonequilibrium steady states
(PTEP Editors' Choice)
Prog. Theor. Exp. Phys. 2023, 083A01 (2023).
A groundbreaking study reveals a new time-local fluctuation theorem using machine learning, revolutionizing our understanding of deterministic nonequilibrium steady state systems.
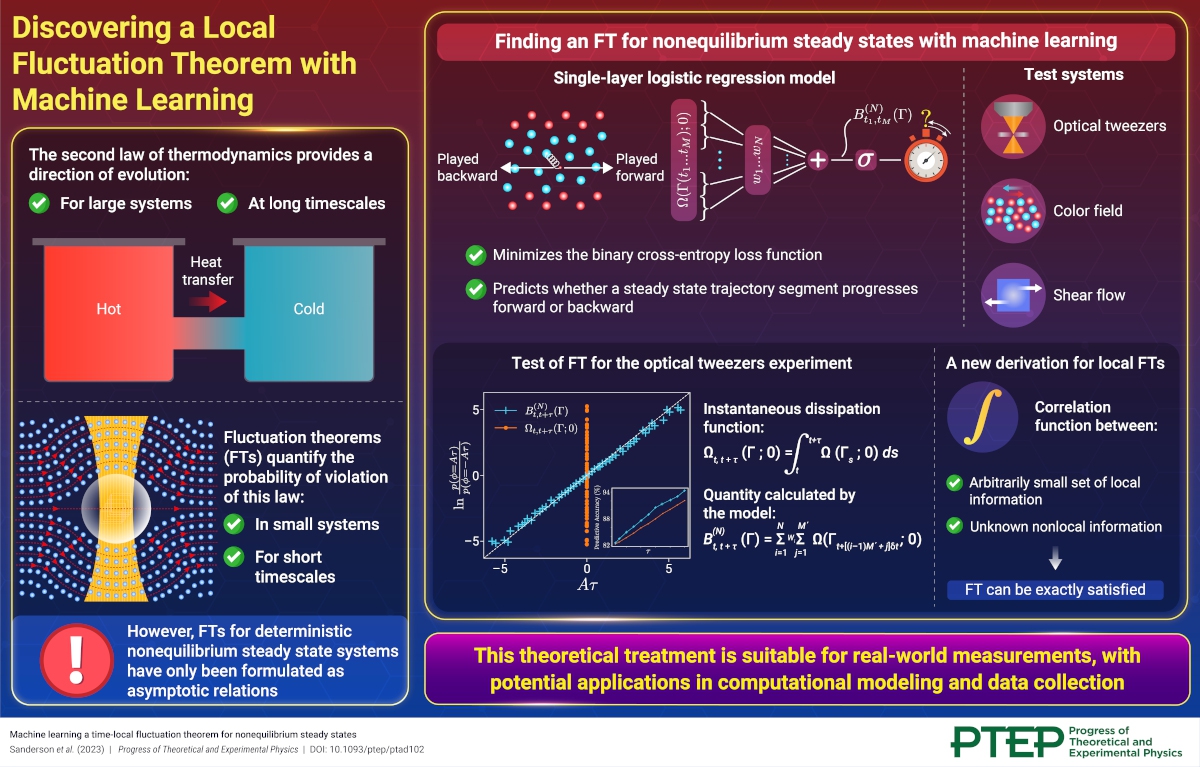
The second law of thermodynamics states that for large systems, there is a direction associated with their evolution over large time scales. However, in the realm of nonequilibrium dynamics, especially where a system is driven far from equilibrium, understanding and quantifying thermodynamic relations is challenging.
In this regard, fluctuation theorems (FTs), which quantify the thermodynamic reversibility of a system in terms of a dissipation function, have emerged as an essential tool for characterizing the probability of observing events in small systems during short timescales that violate the second law of thermodynamics. However, these theorems have only been formulated for a limited class of systems.
Consequently, our understanding of deterministic nonequilibrium steady states—a system with unknown phase space distribution for which only asymptotic FTs hold— remains limited. This is because all attempts to evaluate their dissipation function require either the trajectory segment of interest to be relatively long or information about the entire trajectory around that segment.
In this study, we addressed this long-standing problem in statistical mechanics by utilizing a simple machine learning model. The model was trained to minimize the binary cross-entropy loss function and discern whether a segment of a steady state trajectory progresses forward or backward in time. This yielded a function that satisfied an FT and relied only upon the information within the segment of interest.
Remarkably, this time-local FT is applicable even to extremely short trajectory segments and holds true for systems far from equilibrium as well as for various nonequilibrium dynamics, surpassing all previously known theoretical approximations.
We further demonstrated that any model reaching the global minimum of the binary cross-entropy loss function must be a well-calibrated predictor of time’s arrow and satisfy an FT. This leads us to a new derivation for local-time FTs: an FT can be exactly satisfied for an arbitrarily small set of local information provided the correlation function between that information and the unknown, non-local information is known.
Since our theoretical treatment is applicable to feasible real-world measurements, which only capture information from a local region and for a finite duration, it could potentially have wide-ranging applications in computational modeling as well as real-world data collection.
Consequently, this study could eventually lead to an enhanced understanding of nonequilibrium steady state systems across diverse fields, including fluid dynamics, heat transfer, semiconductor operation, ion transport, biology, and climate modeling.
Machine learning a time-local fluctuation theorem for nonequilibrium steady states
(PTEP Editors' Choice)
Prog. Theor. Exp. Phys. 2023, 083A01 (2023).
Share this topic
Fields
Related Articles
-
Solving a Stochastic Differential Equation is Solving a Mean-Field Quantum Spin System
Statistical physics and thermodynamics
Mathematical methods, classical and quantum physics, relativity, gravitation, numerical simulation, computational modeling
Magnetic properties in condensed matter
2024-5-16
The replica method maps matrix-valued geometric Brownian motion to a mean-field quantum spin system. This correspondence makes it possible to obtain an exact solution for matrix-valued geometric Brownian motion.
-
Thermodynamic Property of a CMOS Device beyond Landauer Limit
Statistical physics and thermodynamics
Electronic transport in condensed matter
Cross-disciplinary physics and related areas of science and technology
2024-1-23
Focusing on a CMOS NAND GATE operating in a sub-threshold region, the thermodynamic cost of computation was analyzed in relation to input/output voltages surpassing the Landauer limit.
-
Exploring Recent Advances in the Physics of Biofluid Locomotion
Measurement, instrumentation, and techniques
Cross-disciplinary physics and related areas of science and technology
Electromagnetism, optics, acoustics, heat transfer, and classical and fluid mechanics
Statistical physics and thermodynamics
Mathematical methods, classical and quantum physics, relativity, gravitation, numerical simulation, computational modeling
Structure and mechanical and thermal properties in condensed matter
2023-12-8
This Special Topics Edition of the JPSJ describes the latest advances in the field of biofluid locomotion, shedding light on the underlying physics behind the movement of organisms that swim and fly.
-
Net-Pattern of Muskmelon Rind Favors a Common Mathematical Law
Cross-disciplinary physics and related areas of science and technology
Statistical physics and thermodynamics
2023-1-12
The surface of muskmelon is covered with a fine mesh-like net-pattern. The geometric features of the fine mesh appear unique for each individual, but hide an unexpected mathematical rule.
-
High Magnetic Field as a Tool for Discovery in Condensed Matter Physics
Magnetic properties in condensed matter
Dielectric, optical, and other properties in condensed matter
Electronic transport in condensed matter
Structure and mechanical and thermal properties in condensed matter
Electron states in condensed matter
Electronic structure and electrical properties of surfaces and nanostructures
Measurement, instrumentation, and techniques
2022-12-13
The Journal of the Physical Society of Japan highlights in this special topic recent advances in modern physics that have been realized with the generation of pulsed high magnetic fields.