Solving a Stochastic Differential Equation is Solving a Mean-Field Quantum Spin System
© The Physical Society of Japan
This article is on
J. Phys. Soc. Jpn.
92,
114001
(2023)
.
The replica method maps matrix-valued geometric Brownian motion to a mean-field quantum spin system. This correspondence makes it possible to obtain an exact solution for matrix-valued geometric Brownian motion.
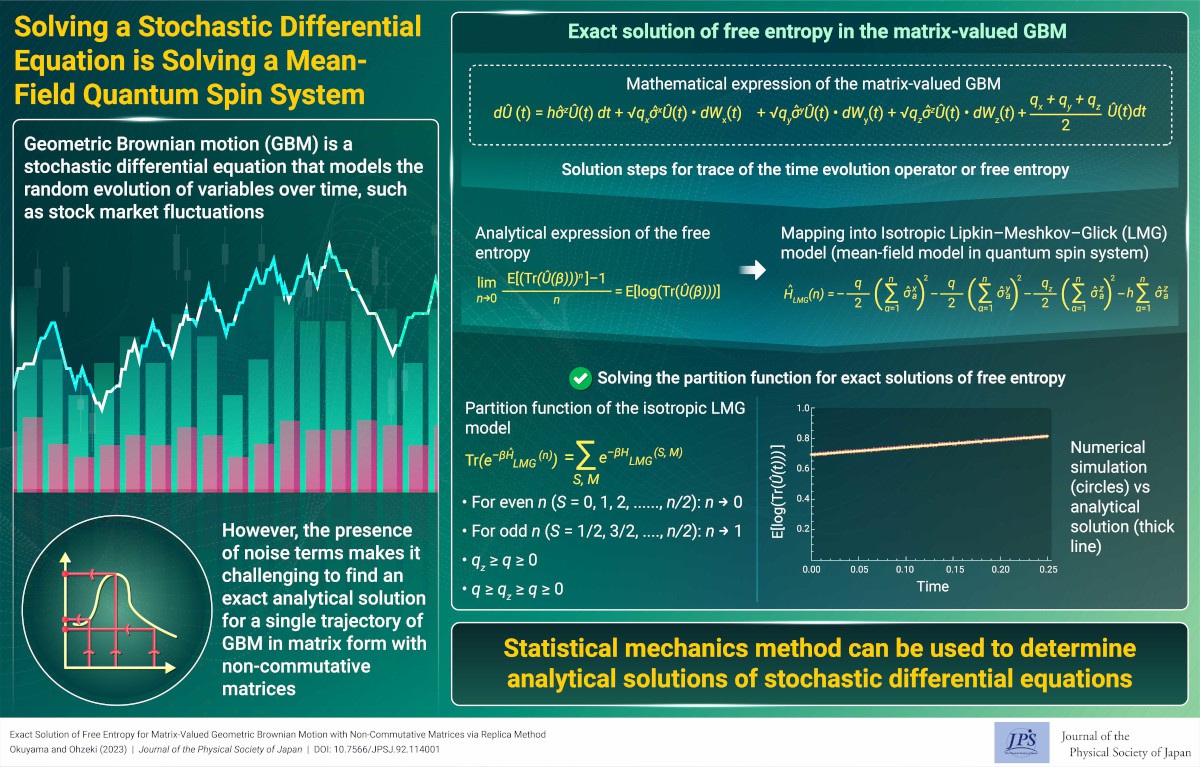
Stochastic differential equations are differential equations where stochastic terms are introduced. Stochastic differential equations were originally introduced by Einstein in research on Brownian motion and are now used not only in mathematics and physics, but also in various fields such as financial engineering. In many cases, solving stochastic differential equations analytically is extremely difficult compared to solving ordinary differential equations, and solving stochastic differential equations numerically also incurs a much higher cost than solving ordinary differential equations.
Our research attempts to obtain analytical solutions for stochastic differential equations. Geometric Brownian motion is one of the most famous stochastic differential equations for which analytical solutions have been obtained. Geometric Brownian motion is used in financial engineering option pricing models. Geometric Brownian motion can be regarded as an ordinary first-order linear homogeneous differential equation, in which the coefficients are replaced by stochastic noise. The exact solution of the geometric Brownian motion can be easily obtained in the same manner as an ordinary first-order homogeneous linear differential equation can be easily solved.
What happens when we consider multivariable geometric Brownian motion? In the case of ordinary differential equations, multivariable first-order homogeneous linear differential equations can be solved by diagonalizing the matrices. However, for multivariable geometric Brownian motion, this problem becomes extremely difficult, and when there is more than one stochastic noise, multivariable geometric Brownian motion cannot be solved by diagonalization. In other words, it has been believed that it is impossible to obtain an exact solution for multivariable geometric Brownian motion.
Under these circumstances, we considered a 2x2 matrix-valued geometric Brownian motion to be the simplest and most nontrivial multivariable geometric Brownian motion. As explained above, it is difficult to solve the 2x2 matrix-valued geometric Brownian motion using the conventional method. Instead, we applied the replica method developed in the spin-glass theory of statistical physics (which is closely related to the Nobel Prize in Physics awarded to Parisi in 2021). The replica method analyzes a model with randomness by mapping it onto an effective model without randomness.
Using the replica method, we found that the time-evolution operator of the matrix-valued geometric Brownian motion can be mapped to the partition function of a mean-field quantum spin system called the Lipkin-Meshkov-Glick model, which was originally proposed in nuclear physics. Furthermore, by analyzing the partition function of the Lipkin-Meshkov-Glick model, we succeeded in obtaining analytical solutions for various quantities of matrix-valued geometrical Brownian motion.
In summary, by analyzing a mean-field quantum spin system, we obtained exact solutions for various quantities of matrix-valued geometric Brownian motion that was previously thought to be unsolvable. Our results imply that there is a close connection between matrix-valued geometric Brownian motion and a mean-field quantum spin system, which, at first glance, have nothing to do with each other.
Written by Manaka Okuyama on behalf of all authors
J. Phys. Soc. Jpn.
92,
114001
(2023)
.
Share this topic
Fields
Related Articles
-
Traffic Signal Optimization using Quantum Annealing
Mathematical methods, classical and quantum physics, relativity, gravitation, numerical simulation, computational modeling
2025-2-25
This paper introduces a QUBO model for traffic signal optimization on real-world maps. Using D-Wave quantum annealing and SUMO simulations, the model reduces vehicle waiting times, but underperforms classical solvers.
-
Symmetry and AI: Building the Future of Physics Simulations
Magnetic properties in condensed matter
Measurement, instrumentation, and techniques
2025-2-18
Generative artificial intelligence (AI) has gained considerable attention in scientific fields. By embedding physical symmetry into AI before training, we created a faster and lighter model. Scaling improves the accuracy and unlocks the potential of physics research and applications.
-
Hyperuniform and Multifractal States in Bosonic Quasicrystalline Systems
Statistical physics and thermodynamics
Structure and mechanical and thermal properties in condensed matter
2025-2-10
Quantum states can be categorized as hyperuniform or multifractal based on electronic characteristics. This study demonstrates that bosonic quasicrystalline systems exhibit hyperuniform or multifractal quantum states.
-
Triangular Lattice Magnet GdGa2: Spin Cycloids and Skyrmions
Cross-disciplinary physics and related areas of science and technology
Electronic transport in condensed matter
Magnetic properties in condensed matter
2025-2-3
Careful measurements were conducted on the hexagonal magnet GdGa2 to reveal the experimental signatures of ultrasmall spin cycloids and of a potential Néel-type skyrmion lattice phase induced by a magnetic field.
-
Spin-Spin Interaction Mediated by Rotational Lattice Vibrations
Magnetic properties in condensed matter
Structure and mechanical and thermal properties in condensed matter
2025-1-24
This study predicts the presence of spin-spin interactions mediated by the angular momentum of lattice vibrations, which can be long-range.