Discovering a Local Fluctuation Theorem with Machine Learning
© The Physical Society of Japan
This article is on
Machine learning a time-local fluctuation theorem for nonequilibrium steady states
(PTEP Editors' Choice)
Prog. Theor. Exp. Phys.
2023,
083A01
(2023)
.
A groundbreaking study reveals a new time-local fluctuation theorem using machine learning, revolutionizing our understanding of deterministic nonequilibrium steady state systems.
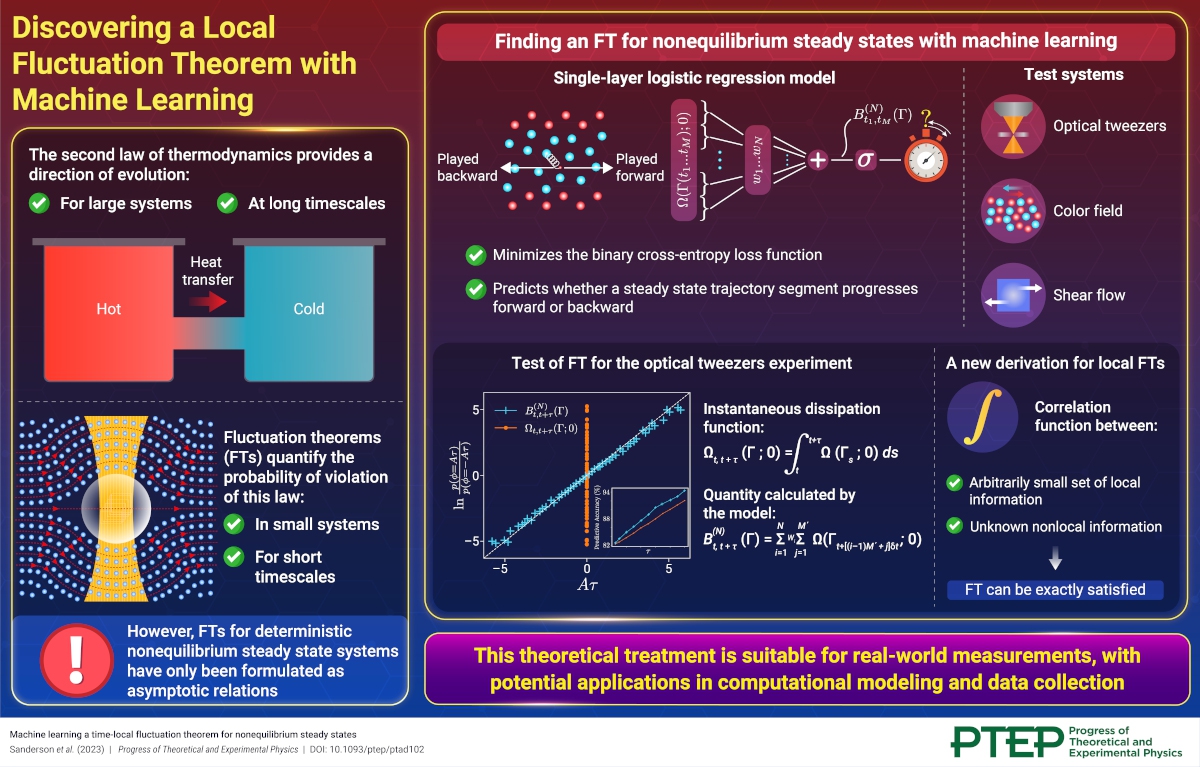
The second law of thermodynamics states that for large systems, there is a direction associated with their evolution over large time scales. However, in the realm of nonequilibrium dynamics, especially where a system is driven far from equilibrium, understanding and quantifying thermodynamic relations is challenging.
In this regard, fluctuation theorems (FTs), which quantify the thermodynamic reversibility of a system in terms of a dissipation function, have emerged as an essential tool for characterizing the probability of observing events in small systems during short timescales that violate the second law of thermodynamics. However, these theorems have only been formulated for a limited class of systems.
Consequently, our understanding of deterministic nonequilibrium steady states—a system with unknown phase space distribution for which only asymptotic FTs hold— remains limited. This is because all attempts to evaluate their dissipation function require either the trajectory segment of interest to be relatively long or information about the entire trajectory around that segment.
In this study, we addressed this long-standing problem in statistical mechanics by utilizing a simple machine learning model. The model was trained to minimize the binary cross-entropy loss function and discern whether a segment of a steady state trajectory progresses forward or backward in time. This yielded a function that satisfied an FT and relied only upon the information within the segment of interest.
Remarkably, this time-local FT is applicable even to extremely short trajectory segments and holds true for systems far from equilibrium as well as for various nonequilibrium dynamics, surpassing all previously known theoretical approximations.
We further demonstrated that any model reaching the global minimum of the binary cross-entropy loss function must be a well-calibrated predictor of time’s arrow and satisfy an FT. This leads us to a new derivation for local-time FTs: an FT can be exactly satisfied for an arbitrarily small set of local information provided the correlation function between that information and the unknown, non-local information is known.
Since our theoretical treatment is applicable to feasible real-world measurements, which only capture information from a local region and for a finite duration, it could potentially have wide-ranging applications in computational modeling as well as real-world data collection.
Consequently, this study could eventually lead to an enhanced understanding of nonequilibrium steady state systems across diverse fields, including fluid dynamics, heat transfer, semiconductor operation, ion transport, biology, and climate modeling.
Machine learning a time-local fluctuation theorem for nonequilibrium steady states
(PTEP Editors' Choice)
Prog. Theor. Exp. Phys.
2023,
083A01
(2023)
.
Share this topic
Fields
Related Articles
-
Qualitative Changes in Kinetic Pathways Driven by Hydrodynamic Interactions in Dense Colloidal Suspensions
Cross-disciplinary physics and related areas of science and technology
Statistical physics and thermodynamics
Structure and mechanical and thermal properties in condensed matter
2025-4-18
Even in dense colloidal suspensions, where long-range hydrodynamic interactions are screened, near-field hydrodynamic interactions qualitatively influence the selection of kinetic pathways.
-
Overcoming Phase Transitions for Faster Quantum Annealing
Statistical physics and thermodynamics
2025-3-27
This study presents an innovative method to address the problem of phase transitions in quantum annealing, resulting in an exponential speedup of the process.
-
Exploring the Vibrant Interplay of Machine Learning and Physics
Cross-disciplinary physics and related areas of science and technology
Electron states in condensed matter
Elementary particles, fields, and strings
Mathematical methods, classical and quantum physics, relativity, gravitation, numerical simulation, computational modeling
Statistical physics and thermodynamics
Superconductivity
2025-3-13
This Journal of the Physical Society of Japan Special Topics edition explores how physics and machine learning complement each other and can solve unresolved problems in physics.
-
Symmetry and AI: Building the Future of Physics Simulations
Magnetic properties in condensed matter
Measurement, instrumentation, and techniques
2025-2-18
Generative artificial intelligence (AI) has gained considerable attention in scientific fields. By embedding physical symmetry into AI before training, we created a faster and lighter model. Scaling improves the accuracy and unlocks the potential of physics research and applications.
-
Bayesian Insights into X-ray Laue Oscillations: Quantitative Surface Roughness and Noise Modeling
Measurement, instrumentation, and techniques
Structure and mechanical and thermal properties in condensed matter
2025-2-14
This study adopts Bayesian inference using the replica exchange Monte Carlo method to accurately estimate thin-film properties from X-ray Laue oscillation data, enabling quantitative analysis and appropriate noise modeling.