Symmetry and AI: Building the Future of Physics Simulations
© The Physical Society of Japan
This article is on
Self-Learning Monte Carlo with Equivariant Transformer
(JPSJ Editors' Choice)
J. Phys. Soc. Jpn.
93,
114007
(2024)
.
Generative artificial intelligence (AI) has gained considerable attention in scientific fields. By embedding physical symmetry into AI before training, we created a faster and lighter model. Scaling improves the accuracy and unlocks the potential of physics research and applications.
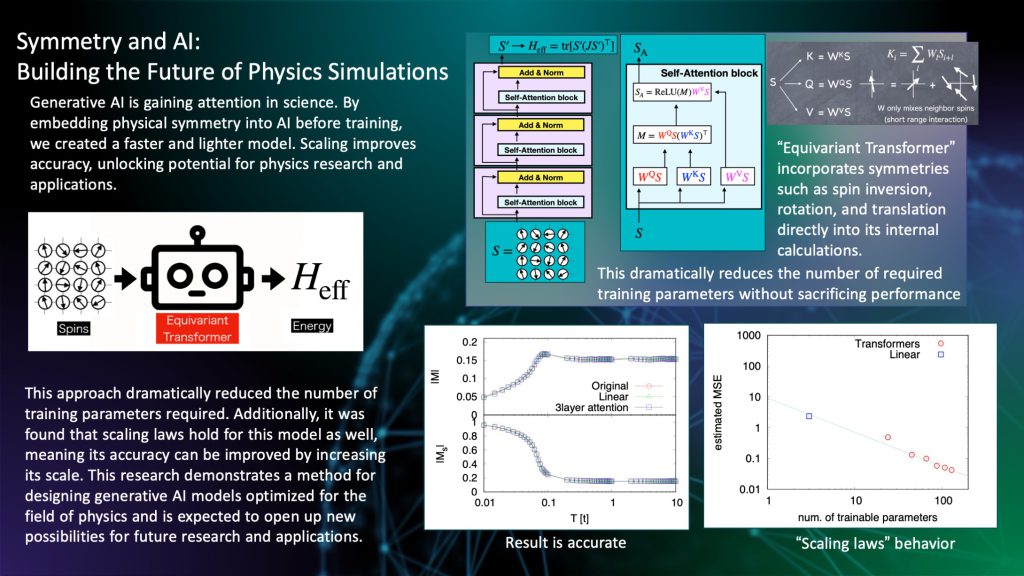
Generative AI automatically creates text, images, and music, converting ideas into tangible outputs. It has become an essential tool for creativity and problem-solving, with applications expanding in everyday life, education, and work. Innovations such as OpenAI’s ChatGPT and Google Gemini have further boosted their popularity. Generative AI and machine learning technologies have seen explosive growth in recent years and are now indispensable in scientific research and development. In physics, AI and machine learning are recognized as revolutionary tools, following traditional methods such as paper, pencil, and computers. For example, molecular dynamics simulations that track the motion of atoms over time often require highly precise calculations that account for quantum mechanical effects. These simulations sometimes take years even when supercomputers are used. However, by replacing computationally heavy parts with neural-network-based machine learning simulations, calculations can be accelerated by more than 1,000 times. This reduced the three-year simulation time to one day. Such advancements allow researchers to screen materials such as new battery components much faster, saving both time and cost during synthesis and experimentation. Generative AI, which is one of the most successful AI technologies, has immense potential for transforming physics. However, conventional generative AI models, such as those used for natural language processing, often contain billions of parameters. Although effective in their domains, they are computationally expensive and unsuitable for direct use in physical simulations. Large models also face the “black box” issue, where their processes are so complex that humans struggle to interpret the underlying physics. However, machine learning models specifically designed for physics are often too simple and lack the complexity required for high-accuracy simulations. Balancing simplicity and complexity is a significant challenge. A solution lies in leveraging symmetry, a key principle in physics, where an object remains unchanged under certain transformations, such as reflection, rotation, or translation. Symmetry explains fundamental principles, such as energy and momentum conservation. We recently developed an “Equivariant Transformer,” an AI model that incorporates these symmetries into its internal structure. By doing so, they drastically reduce the number of training parameters required while maintaining accuracy. Additionally, this model follows “scaling laws,” meaning its performance improves as the model size increases, just like in large language models.
This breakthrough demonstrated how generative AI can be adapted to physics by embedding symmetry principles and creating fast, accurate, and interpretable models. These advancements can lead to more efficient simulations, trustworthy AI systems, and new discoveries in physics, thus paving the way for exciting research opportunities.
(Written by Yuki Nagai on behalf of all authors)
Self-Learning Monte Carlo with Equivariant Transformer
(JPSJ Editors' Choice)
J. Phys. Soc. Jpn.
93,
114007
(2024)
.
Share this topic
Fields
Related Articles
-
Bayesian Insights into X-ray Laue Oscillations: Quantitative Surface Roughness and Noise Modeling
Measurement, instrumentation, and techniques
Structure and mechanical and thermal properties in condensed matter
2025-2-14
This study adopts Bayesian inference using the replica exchange Monte Carlo method to accurately estimate thin-film properties from X-ray Laue oscillation data, enabling quantitative analysis and appropriate noise modeling.
-
Exploring Materials without Data Exposure: A Bayesian Optimizer using Secure Computation
Cross-disciplinary physics and related areas of science and technology
Measurement, instrumentation, and techniques
2025-2-6
Secure computation allows the manipulation of material data without exposing them, thereby offering an alternative to traditional open/closed data management. We recently reported the development of an application that performs Bayesian optimization using secure computation.
-
Triangular Lattice Magnet GdGa2: Spin Cycloids and Skyrmions
Cross-disciplinary physics and related areas of science and technology
Electronic transport in condensed matter
Magnetic properties in condensed matter
2025-2-3
Careful measurements were conducted on the hexagonal magnet GdGa2 to reveal the experimental signatures of ultrasmall spin cycloids and of a potential Néel-type skyrmion lattice phase induced by a magnetic field.
-
Spin-Spin Interaction Mediated by Rotational Lattice Vibrations
Magnetic properties in condensed matter
Structure and mechanical and thermal properties in condensed matter
2025-1-24
This study predicts the presence of spin-spin interactions mediated by the angular momentum of lattice vibrations, which can be long-range.
-
Shaping the Future of Materials Science with Tanabe–Sugano Diagrams
Dielectric, optical, and other properties in condensed matter
Electron states in condensed matter
Electronic structure and electrical properties of surfaces and nanostructures
Magnetic properties in condensed matter
2025-1-21
This special collection published in the Journal of the Physical Society of Japan celebrates 70 Years of Tanabe–Sugano Diagrams, highlighting their continued role in advancing materials with transition metals.